
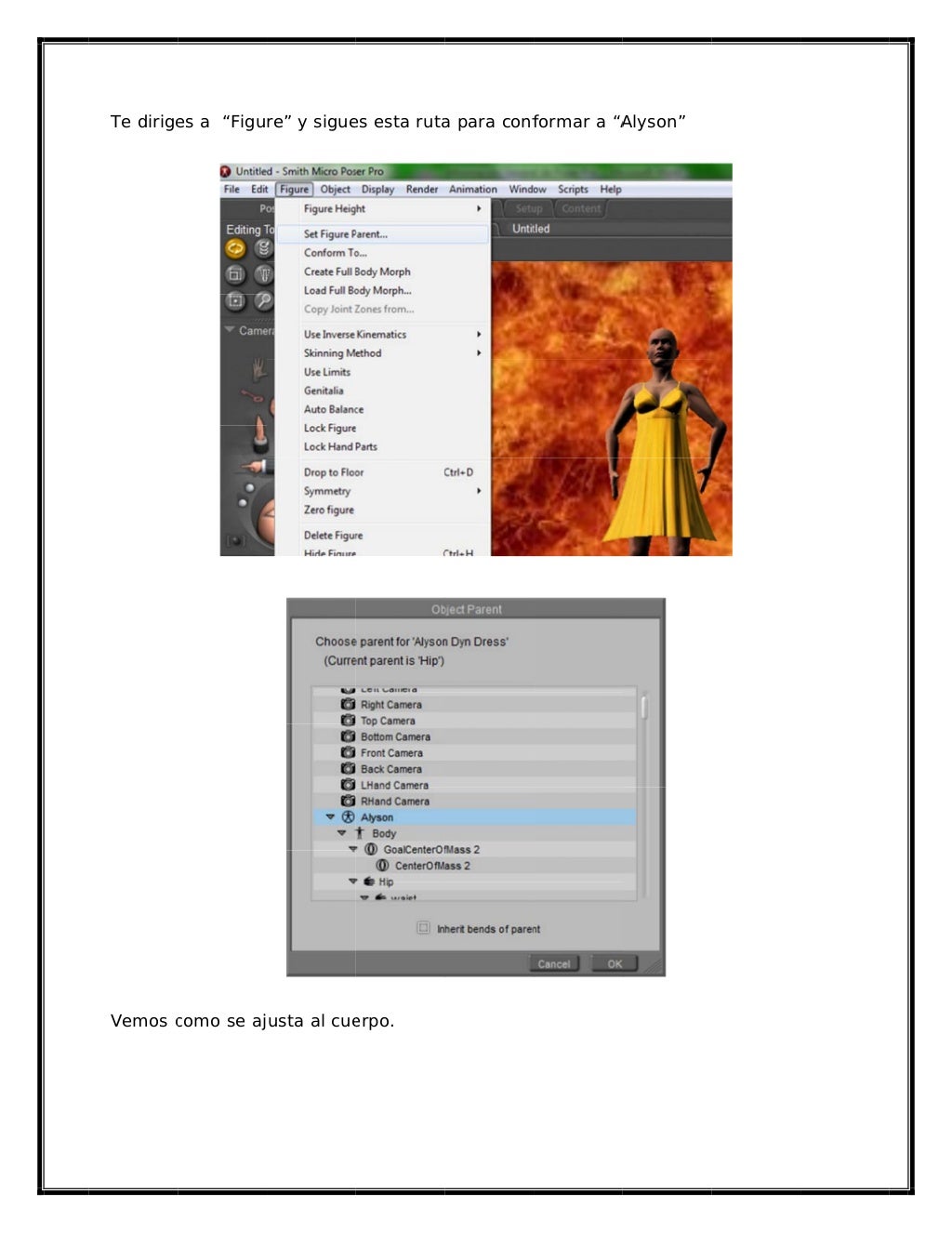
By developing a hierarchical multivariate hidden Markov model with reactive interpolation functionality the system learns the structure of the motion sequences. Then, the data is preprocessed in such a way that would be handled more efficiently. The user's full-body locomotion and the IMU's data are recorded simultaneously. To solve such a complex problem, the presented method is divided into several steps. This process can be characterized by its difficulty because of the need to reconstruct a high number of degrees of freedom (DOFs) from a very low number of DOFs. This paper presents a method of reconstructing full-body locomotion sequences for virtual characters in real-time, using data from a single inertial measurement unit (IMU).
#Poser pro 2012 descargar gratis code#
DIP-IMU and the code are available for research purposes. We quantitatively evaluate our approach on multiple datasets and show results from a real-time implementation. To evaluate our method, we recorded DIP-IMU, a dataset consisting of $10$ subjects wearing 17 IMUs for validation in $64$ sequences with $330\,000$ time instants this constitutes the largest IMU dataset publicly available. At test time, we deploy the network in a sliding window fashion, retaining real time capabilities. A bi-directional RNN architecture leverages past and future information that is available at training time. To learn from sufficient data, we synthesize IMU data from motion capture datasets. To address this important limitation, we learn the temporal pose priors using deep learning. Third, modeling temporal dependencies through non-linear optimization has proven effective in prior work but makes real-time prediction infeasible.

Second, capturing IMU data in conjunction with ground-truth poses is expensive and difficult to do in many target application scenarios (e.g., outdoors). First, the problem is severely under-constrained as multiple pose parameters produce the same IMU orientations.
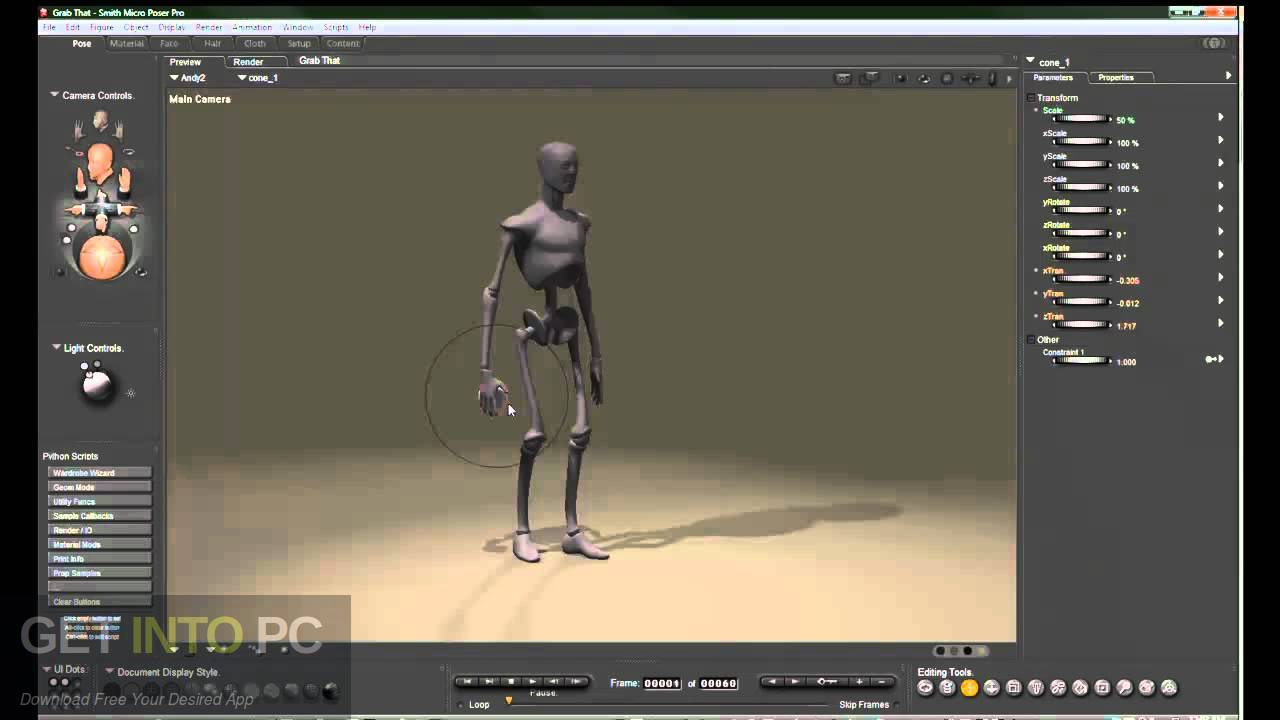
In doing so, we address several difficult challenges.
#Poser pro 2012 descargar gratis full#
We demonstrate a novel deep neural network capable of reconstructing human full body pose in real-time from 6 Inertial Measurement Units (IMUs) worn on the user's body.
